Featured Resource
Beyond VPNs: A Deep Dive into Microsoft’s Remote Access Options
Join us as we examine the various technology options and use cases for remote access. Increasingly distributed work environments have created both opportunities and challenges... Learn more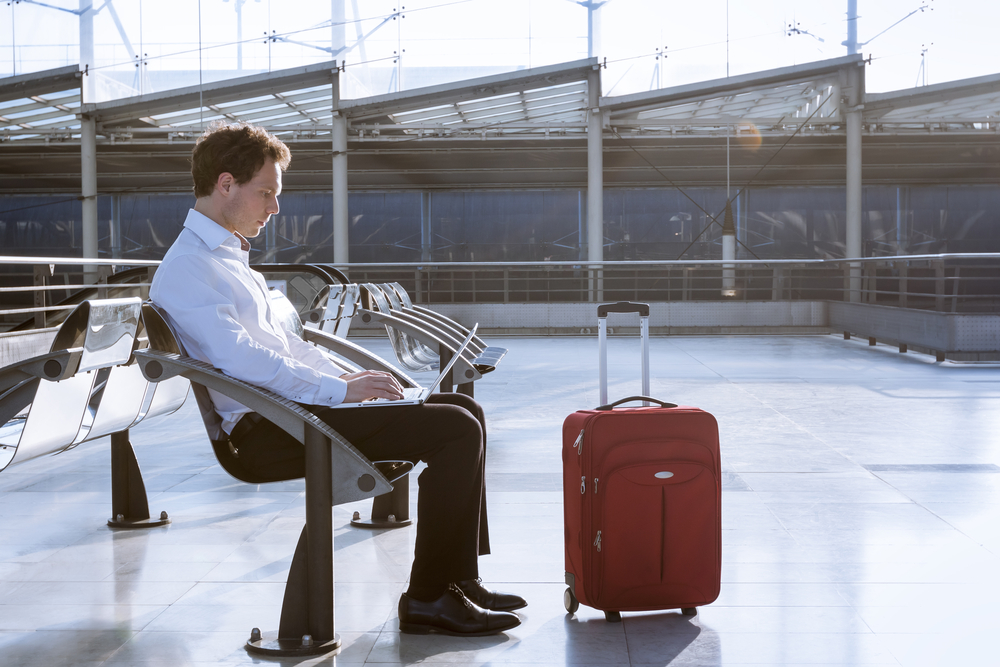
Loading...